Of the many exciting fields in computer science, Machine Learning may be the most immediately applicable to industry. This subset of AI research is already changing the landscape of cybersecurity, medicine, animal research, and anything involving big data. With innovation happening every day at MIT and beyond, this technology has much to offer both consumers and business leaders.
How will Machine Learning affect you?
Agenda
Welcome | 10:00am
- Lori Glover, Managing Director, MIT CSAIL Global Strategic Alliances
Keynote | 10:05-10:35am
- Professor Daniela Rus, Director at MIT CSAIL | Professor, MIT EECS
More Efficient Machine Learning Panel | 10:35-11:20am
- Computational limits of Deep Learning: Real-time Inference on Edge Devices via Adaptive Model Streaming
- Mohammad Alizadeh, Professor, MIT EECS & Mehrdad Khani Shirkoohi, PhD Student, MIT CSAIL
- Mining the Pareto Frontier for More Efficient ML models
- Karima Ma, PhD Student, MIT CSAIL
- MLA: Data-Efficient, Debiased, Social Machine Learning
- Boris Katz, Principal Research Scientist, MIT CSAIL & Andrei Barbu, Research Scientist, MIT CSAIL
- Industry Panel (11:20-11:35am)
- Steve Whittaker, Chief Research Scientist & Head of Strategic US University Research Partnerships, BT
- Vishal Gossain, Practice Leader, Risk Analytics and Strategy, EY
- Ali Payani, Senior AI Researcher, Cisco
Leveraging Data for Better Machine Learning Applications Panel | 12:05-12:50pm
- LANCET: Labeling Complex Data at Scale
- Lei Cao, Research Scientist, MIT CSAIL
- Few-Shot Learning with Large Language Models: Efficiently Adapting to New Domains
- David Sontag, Professor MIT EECS & Yoon Kim, Professor MIT EECS
- Off-the-Shelf Natural Language Processing of Deep Networks
- Jacob Andreas, Professor, MIT EECS
- Industry Panel (12:50-1:05pm)
- Steve Whittaker, Chief Research Scientist & Head of Strategic US University Research Partnerships, BT
- Vishal Gossain, Practice Leader, Risk Analytics and Strategy, EY
- Ali Payani, Senior AI Researcher, Cisco
Responsible Machine Learning | 2:20-3:05pm
- Responsible AI: Can Dropping a Little Data Change Results and Conclusions?
- Tamara Broderick, Professor, MIT EECS
- Uncovering Unexpected Behavior of ML Models Pre-Deployment
- Saachi Jain, PhD Student, MIT CSAIL
- Bias-free ML, Responsible ML, Detecting Bias, and Moving toward Explainable AI
- Amar Gupta, Research Scientist, MIT CSAIL
- Industry Panel (3:05-3:20pm)
- Steve Whittaker, Chief Research Scientist & Head of Strategic US University Research Partnerships, BT
- Vishal Gossain, Practice Leader, Risk Analytics and Strategy, EY
- Ali Payani, Senior AI Researcher, Cisco
Wrap & Close | 3:20-3:35pm
- Lori Glover, Managing Director, MIT CSAIL Global Strategic Alliances
To learn more about MachineLearningApplications@CSAIL, visit: https://bit.ly/3RVAlXh. Sign up for our mailing list to learn more about the conference and MLA@CSAIL.
Machine learning is everywhere. It’s used to improve the algorithms generating social media recommendations or online ads. It’s helping us understand animal research and climate change. It creates the chatbots we speak to and the voice assistants we use every day like Alexa, Siri, and Google. Machine learning is changing healthcare, improving autonomous cars, developing new batteries, assisting digital manufacturing, analyzing investment opportunities, even well on its way to understanding human language. And according to business leaders, this is only the beginning.
What is machine learning?
Machine Learning (ML) is a subset of Artificial Intelligence (AI) which focuses on creating systems that use data to improve accuracy in much the same way that humas learn new skills or information. In a world overflowing with data (customer profiles, buying habits, stock trends, genomes, etc.), ML is exciting because it’s all about using data to generate smarter algorithms, feeding specific information into a computer to create useful outputs. If trained properly, these ‘educated’ machines can read enormous volumes of data and extract information that might never be possible otherwise.
There are, broadly speaking, four types of ML: supervised learning, in which scientists give the algorithm labeled training data with well-defined variables; unsupervised learning, when the machine is fed unlabeled raw data and asked to extract information out of it; semi-supervised learning, a mix of the first two; and reinforcement learning, where the machine is trained not with data sets but trial and error. Most applications of ML involve some combination of these methods, and ML often intersects with other exciting fields of computer science research, such as deep learning and robotics. So, while these categories offer a general insight into how ML research is done, the field itself is nowhere near that simple.
What are the current problems in machine learning?
It wouldn’t be an exciting area of research if there weren’t problems yet to be solved, and ML is no exception.
Right now, the biggest obstacle to effective ML is finding or creating good data sets to train with. The problem is that the algorithm is only as strong as the data fed to it, and that often leads to a whole host of issues. For example, in predicting who might be accepted into law school, an algorithm trained with historical research is likely to give women a lower score because of their historically underrepresented status. Unchecked, real-world bias and discrimination will influence the data, which could have disastrous effects on the machines that are trained with them. If we plan to use these algorithms for consequential decisions such as job recruiting, then we must strive to make them fair and just. Another issue in machine learning is public perception. One doesn’t have to dig very deep to find a viral article about job replacement, technological singularities, or privacy concerns. Innovation is exciting, but it doesn’t happen in a vacuum, and the consensus on AI and ML will affect legislation, policy, and business strategies going forward. Therefore, it’s important to make sure the public understands the truth about ML and what it promises to do for humanity.
Why should you care about it?
AI—ML more specifically—isn’t just a headline. It’s a world-changing technology with far-reaching ramifications. It will impact business, education, investing, transportation, and so many other avenues of life and commerce.
Understanding ML is more than just knowing the terms. It’s also knowing how and when to leverage ML, what the drawbacks are, what does skilled work involve, etc. It’s a complex field with equally complex challenges, where the difference between learning from the experts and learning from Google could mean the difference between a neural network that does what you want and one that fails or worse, causes harm. Getting the right information has never been more important. That’s where CSAIL comes in.
What is MachineLearningApplications@CSAIL?
Launched in fall of 2020, the MachineLearningApplications@CSAIL initiative was created by the CSAIL Alliances team to bring industry and research together. Through this program, key industry members fund ML research at CSAIL relating to specific problems they’re facing. This collaboration between scientists and businesses benefits both sides and leads to the kind of problem-solving that will affect the entire field of AI research. Currently, the faculty involved in this initiative are tackling questions like bias in ML, privacy-accuracy tradeoffs, differentiable programming, automated interaction design, and natural language processing. Their cutting-edge work is shaping the development of ML practices all over the world. Whether or not you belong to a Fortune 500 company, you can still participate in this initiative by coming to our public conference and learning about the pioneering work CSAIL faculty are doing in ML with the help of CISCO, SAP, Arrow Electronics, Capital One, and the Ahold Delhaize Company.
Sign up to join our newsletter for information about this and future conferences.
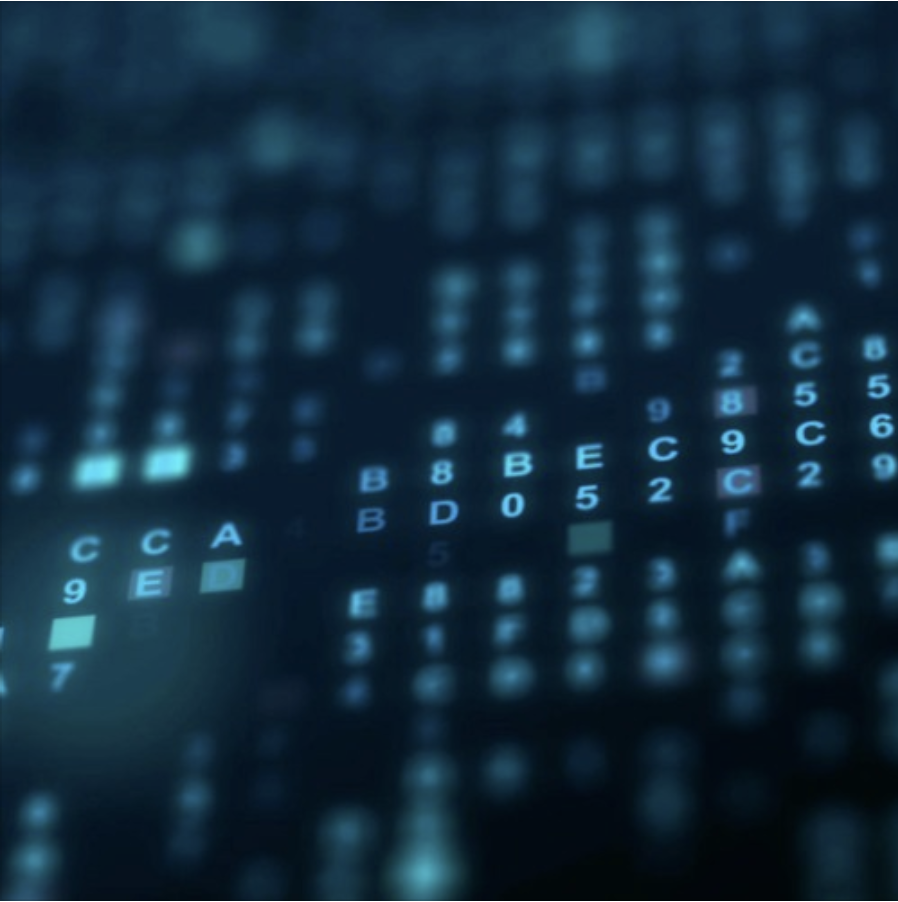
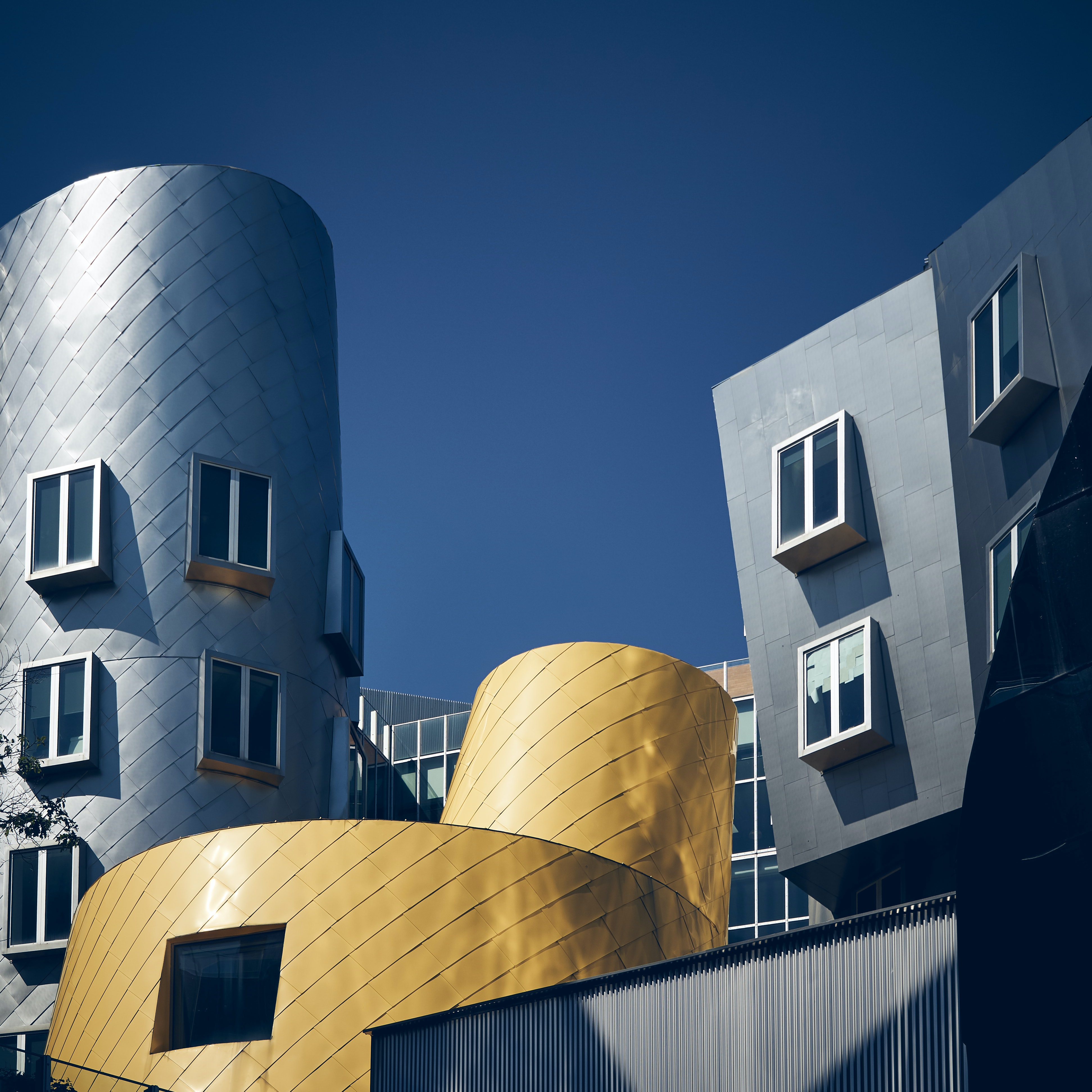