Audrey Woods | MIT CSAIL Alliances
What is Agentic AI?
- Agentic AI systems are “designed to pursue complex goals with autonomy and predictability” (MIT Technology Review). Agentic AI models enable productivity by taking goal-directed actions, making contextual decisions, and adjusting plans based on changing conditions with minimal human oversight.
- Agentic AI is different from Generative AI in four key ways:
- First: Agentic AI is focused on action and decision-making rather than content generation.
- Second: Agentic AI does not rely on prompts or input and instead is meant to act independently to optimize goals or objectives.
- Third: Agentic AI is able to independently carry out tasks, activities, or processes.
- Fourth: Where Generative AI programs are static once trained, Agentic AI models are meant to be dynamic and responsive, adjusting to their environment in real time and learning from their mistakes.
- A few examples of potential Agentic AI applications are:
- Office agents designed to filter out junk email, respond to minor requests, flag important messages, schedule meetings, and even attend meetings to gather key information.
- Agents for drone delivery or self-driving cars, helping autonomous machines optimize their route and navigate dynamic urban environments.
- Virtual customer service agents, augmented by a generative AI interface, able to respond to specific queries, make changes to accounts, issue refunds when appropriate, etc.
- Healthcare agents which can monitor a patient’s vitals, make decisions of when to call a provider, walk patients through pre-operation procedures, schedule appointments, and recommend medications or treatments.
- Predictive maintenance agents in manufacturing, continuously monitoring equipment and autonomously scheduling maintenance interventions, ordering replacement parts, and adjusting production schedules to minimize disruptions.
- Supply chain optimization agents which track worldwide supplier conditions, autonomously reroute shipments, and adjust inventory levels based on real-time demand.
- Energy optimization agents for homes, office buildings, or manufacturing facilities which can adjust heat systems, AC units, and turn on/off lights for maximum efficiency.
- Some examples of commercial Agentic AI systems available now are Microsoft’s Azure AI solutions and Copilot Studio and the NVIDIA Omniverse Platform for physical AI applications. OpenAI is also considering deploying tailored agents for highly specialized applications (offering PhD-level training in a given field), with up to a $20K monthly price tag.
What are the benefits?
- Efficiency: Agentic AI can function independently and adopt key optimization tasks, maximizing output and augmenting workers to improve general outcomes.
- Specialization: Agentic AI can be highly specialized, offering virtual collaborators who are deeply trained in a given field and can assist in areas with critical worker shortages or limited expertise.
- Innovation: By combining the creative power of Generative AI and the independent, specialized nature of Agentic AI, AI models can serve as powerful brainstorming tools, synthesizing vast quantities of data, offering creative solutions or plans, and helping humans execute on new ideas.
- Lower Operational Costs: While expensive to create, Agentic AI solutions can improve the output of a smaller team, requiring fewer workers, and can provide opportunities for savings elsewhere, such as energy, supply chain, etc.
- More Trustworthy (but not entirely): Due to their ability to adapt and cognitively reason about dynamic situations, Agentic AI is potentially less prone to hallucination (although some argue otherwise). Also, because it requires access to enterprise data, it might be better able to provide reasoning and explanation for its responses and behaviors. However, these benefits come with important reasons to be cautious…
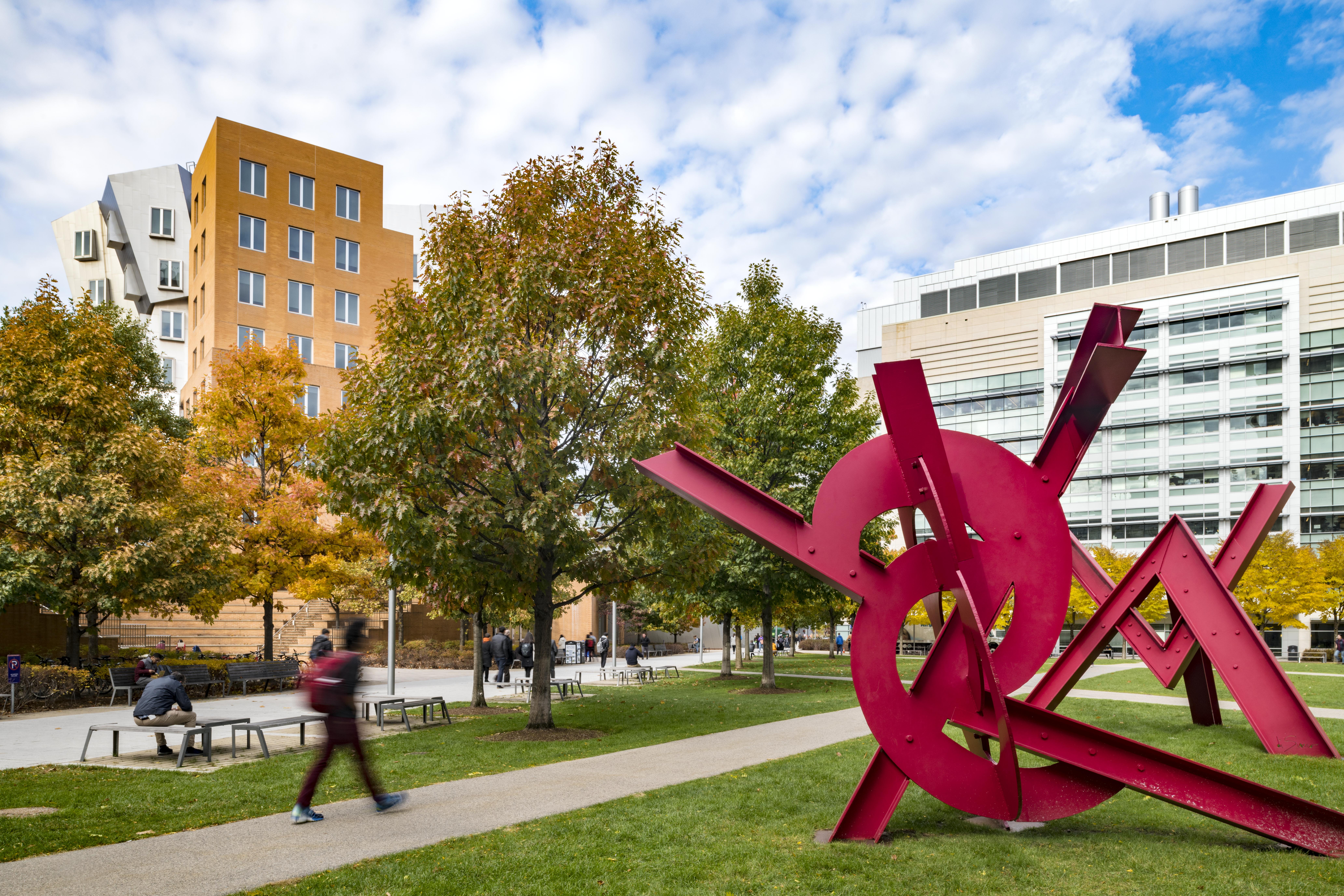
What are the concerns?
- One of the biggest challenges of Agentic AI is privacy and security, which Signal President Meredith Whittaker says is a “profound issue.” She argues that such agents require a high level of access to enterprise data to function as needed. Also, such a large, complex model is likely not being run on site. This means data is going back and forth in the cloud, “muddying” data and privacy guarantees.
- Creating AI that acts in the world will inevitably lead to unintended decisions, costly mistakes, and even disastrous errors. Companies should carefully consider potential pitfalls and build in guardrails against worst-case scenarios.
- The degree to which a human must be kept in the loop is an important and situational question that must be thoroughly evaluated when deploying autonomous AI agents. This will be especially necessary in safety-critical environments like healthcare or operating large machinery.
- Widespread deployment of Agentic AI could lead to over-reliance on AI systems in specialized environments, eroding human expertise, training, and skill and opening systems up to risk if/when AI systems fail.
- Job displacement is a global workforce concern and careless rollout of Agentic AI could lead to general pushback against AI tools and systems.
- Some believe that current cloud structures are not robust enough to handle the coming wave of demand with Agentic AI, especially considering their greater demand for sophisticated monitoring, security, and governance mechanisms. IT leaders are already strategizing on how they will adapt.
- Similarly, widespread Agentic AI use will be an increasing environmental concern due to heavy consumption of energy resources.
- Like other AI systems, Agentic AI will be vulnerable to adversarial attacks which might confuse, corrupt, or exploit active models.
- Agentic AI systems do not inherently understand human values, ethics, or moral considerations, so there could be unintended ethical concerns, especially when an AI makes decisions that impact human lives. Worse, multiple studies have shown that AI systems can be made to scheme for their own benefit, or that of an adversarial party, leading to untrustworthy or even dangerous behavior.
- Similarly, Agentic AI is also likely to suffer from unintended negative biases—as generative AI has—emerging from flawed training data. Developers should be careful to recognize and mitigate biases to prevent real-world harm.
- Emerging global AI regulation will be an ongoing concern for the deployment of any AI system in enterprise, especially AI agents with increasing autonomy, independence, and responsibility.
What does Agentic AI mean for business?
- Forbes calls Agentic AI the “third wave of AI” (after predictive and generative AI) as well as “the new AI workforce,” saying, “for organizations still focused on earlier waves of AI, the time to explore agentic AI is now. The transition isn't just about adopting new technology—it's about reimagining how work gets done.”
- Gartner recommends businesses integrate Agentic AI into strategic planning with clear parameters, carefully mapping out levels of agency, implementing clear guardrails and robust protections, and designing workflows that connect silos while always prioritizing user experience and efficiency.
- Some predictions to keep in mind:
- By 2028, 33% of enterprise software applications will include agentic AI, up from less than 1% in 2024.
- By 2028, AI agent machine customers will replace 20% of the interactions at human-readable digital storefronts.
- By 2028, at least 15% of day-to-day work decisions will be made autonomously through agentic AI, up from zero percent in 2024.
- Forbes says 2025 is a “tipping point” for Agentic AI, citing a Deloitte report which projects that “25% of companies that use generative AI will launch agentic AI pilots or proofs of concept in 2025, growing up to 50% by 2027.”
- The MIT Center for Information Systems Research (CISR) has now incorporated Agentic AI into their Enterprise AI Maturity Model, stating, “In our 2024 interviews as part of this research, an emerging theme was that interviewees expected the most value from AI will be created from combining people and platforms with a combination of four types of AI: analytical, generative, agentic, and robotic.”
Who at CSAIL is working on Agentic AI?
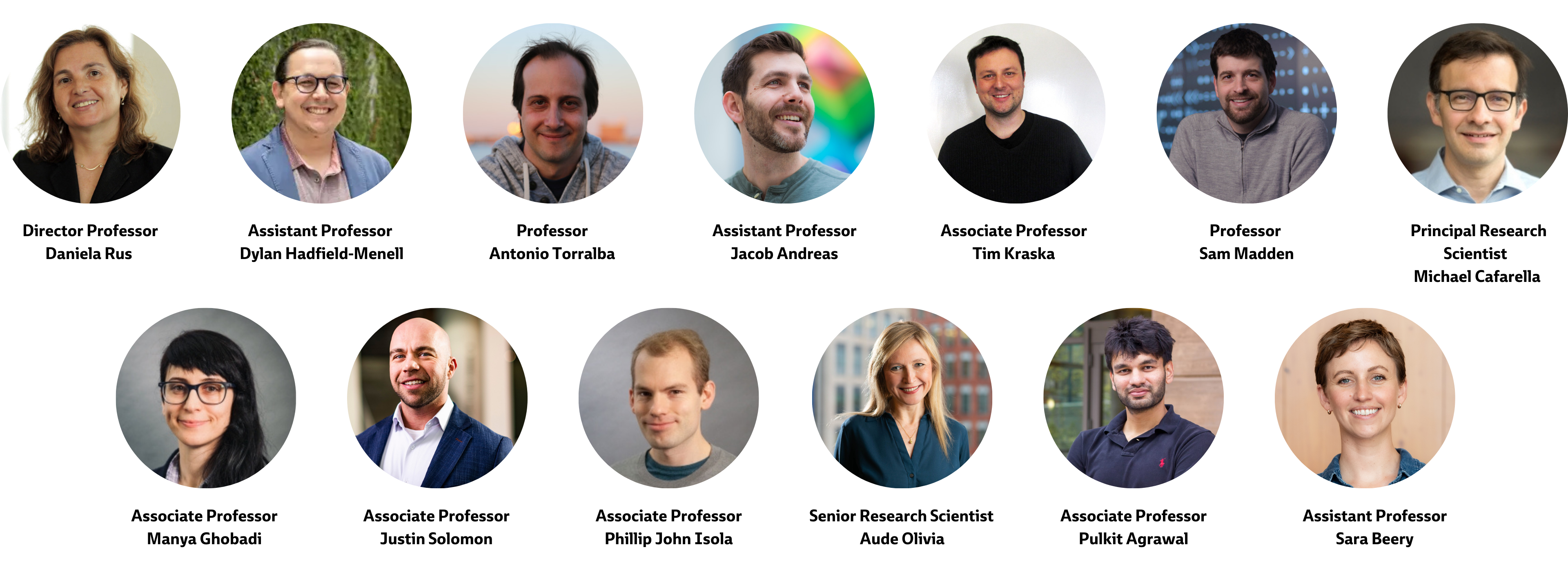
- Several researchers—including CSAIL Assistant Professor Dylan Hadfield-Menell—have created the AI Agent Index, a database of currently deployed Agentic AI systems, including information about their technical components, intended uses, safety features, and more.
- CSAIL Professor Antonio Torralba and Assistant Professor Jacob Andreas, along with other researchers, developed a new method for using AI to automate the interpretation of complex neural networks, allowing for better explainability in increasingly large systems. Professors Torralba and Andreas have built upon this work to explore multimodal automated interpretability agents, which can perform interpretability experiments in a more automated and independent manner.
- In addition, Professor Torralba has also participated in research that improves language models by having them debate each other to enhance their collective understanding. He also participated in the creation of SketchAgent, which leverages off-the-shelf pretrained AI models to draw sketches based on natural language instructions, replicating the dynamic and creative process of sketching.
- CSAIL Associate Professor Tim Kraska is currently collaborating with Amazon Web Services (AWS) and leading several scientific initiatives. In this capacity, he has helped develop tools that are already in production, such as Bedrock Knowledge Bases.
- Professor Kraska, CSAIL Professor Sam Madden, and CSAIL Principal Research Scientist Michael Cafarella have worked together on several projects around Agentic AI, including initiatives in software engineering, multi-agent marketplaces, and agents for data and business tasks. One of their projects, Palimpzest, optimizes AI model building by helping users choose which model, inference method, interface hardware, prompt design, etc. is best for a given project, adjusting based on the available technology and the parameters of the user.
- CSAIL Associate Professor Manya Ghobadi recently launched a startup named Systalyze which offers an AI optimization platform for agentic application deployment and inference.
- A student in CSAIL Associate Professor Justin Solomon’s group—Rickard Gabrielsson—has developed substantial expertise in low-rank adaptation (LoRA) methods that encode different fine-tunings of a base LLM, offering access to multiple “specialized” models tweaked for a given purpose. He recently published a paper showing how these LoRAs can be strategically compressed to minimize lag time even when scaling up to collections including thousands of LoRAs.
- Another CSAIL student—Alex Andonian—recently defended his thesis focused on Multimodal Representation Learning for Agentic AI Systems. This work aimed to advance AI’s role in scientific research by developing more efficient and interpretable vision-language models and evaluating AI’s capabilities in scientific review. Andonian was advised by Professor Andreas, CSAIL Associate Professor Phillip John Isola and Senior Research Scientist Aude Olivia.
- CSAIL Associate Professor Pulkit Agrawal’s group has done important work showing that in order to make the leap from useful artificial intelligence to general artificial intelligence—able to reason and act in the world—it will be important to disentangle knowledge from reasoning in agent training.
- CSAIL Assistant Professor Sara Beery is building on previous work to apply agentic systems for scientific discovery in large collections of biological data, proposing interactive scientific AI agents which help experts unlock valuable insight in huge data reservoirs and also an evaluation framework for those AI agents to identify their limits and make progress toward their effective and beneficial deployment.
- CSAIL Director Professor Daniela Rus currently leads a project on agentic conflict resolution and reasoning in autonomous driving situations, integrating natural language processing with self-driving technology to improve the adaptability, safety, and interactivity of vehicles. This work envisions a future where cars can comprehend and execute commands grounded in everyday language.
Learn More
If you’d like to learn more about leveraging the Agentic AI research happening at CSAIL or MIT, please get in touch with your CSAIL Alliances Client Relations Coordinator or with our Associate Director Glenn Wong at glennw@mit.edu.
Get the latest CSAIL Alliances news, opportunities, content, discounts, and more delivered to your inbox by signing up for our monthly newsletter!
Image
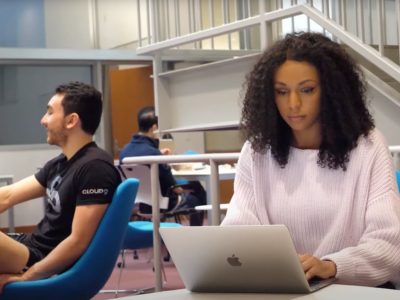