THE CURRENT LANDSCAPE:
Computer science is rich with innovation. Powerful new technologies such as large language models, generative AI programs, cloud computing options, improvements in computer vision, and novel robotic designs are actively impacting how businesses approach their technical futures. To satisfy the expectations of modern consumers and stay competitive in the market, companies strive to make their systems as fast, intelligent, and secure as possible.
The remarkable strides made in machine learning (ML) and artificial intelligence (AI) are accompanied by significant environmental and financial costs. For example, training a single AI model can emit as much carbon as several cars might throughout their entire operational lives. While specific figures can vary widely based on numerous factors, including the energy source, model complexity, and operational efficiency, the broader point remains: the carbon footprint of training sophisticated ML models is substantial. Furthermore, the deluge of data needing to be stored in the cloud compounds these potential environmental impacts. Projections suggest a staggering amount of data creation by 2025, with sustainability becoming a critical concern. The energy consumption and associated carbon emissions from storing and processing these data are immense. As we continue to harness the benefits of ML, it’s imperative to also innovate in energy efficiency and sustainable computing practices. This includes optimizing data center operations, investing in renewable energy sources, and developing more energy-efficient algorithms, all in an effort to mitigate the ecological impact of our technological progress.
Customers, as well as governments, are growing more environmentally conscious and pressure is mounting on companies to make sustainable choices which could prove significant to a company’s bottom line. Aside from the energy cost, the raw price tag on some of these game-changing inventions makes them risky to implement, difficult for smaller businesses to leverage, and may strain company resources. To reap the benefits of these technologies, we need next generation solutions that are fast, intelligent, secure AND sustainable.
INITIATIVE GOALS:
To address this challenge, MIT’s Computer Science and Artificial Intelligence Lab (CSAIL) has created a new initiative that will:
Connect the research in the lab to real-world challenges for which there is no current commercial solution and explore the future impact of next generation software that is efficient, high-performing, and sustainable.
Provide visibility and bring together MIT CSAIL researchers working across disciplines on a variety of next generation software efficiency challenges.
Seed projects that cut across multiple areas addressing next generation software efficiency such as CPU efficiency in datacenters, impact measurement, priorities between efficiency / privacy / security, and ease of use.
Work jointly with industrial partners to create a roadmap of transformative next generation software efficiency technologies that will enable a positive impact in this space.
THE INITIATIVE WILL ADDRESS:
- Performance and efficiency of foundational elements in a post-Moore’s Law world (e.g., operating systems, workload scheduling, utilizing general processors vs accelerators, compiler optimization).
- Compatibility with existing applications and systems for scale and adoption.
- Instrumentation and frameworks for measuring performance, efficiency, and carbon impact in dedicated and distributed workloads.
- Tradeoffs between security, privacy, and efficiency.
- Opportunities to review and improve code embedded into hardware.
- Smarter approaches to model training, such as prefiltering out low-value data.
- Ease of use, wider training of HPC approaches, and relevance in common software development methodologies.
- Creating intelligent systems capable of dynamically allocating computational resources based on workload demands, thereby optimizing energy use without compromising performance.
- Encouraging the development of decentralized and distributed computing networks that can share resources efficiently, reducing redundant energy consumption across systems.
- Leveraging artificial intelligence to continuously optimize computing processes, infrastructure, and energy consumption in real-time, based on predictive analytics and adaptive learning algorithms.
APPLICATIONS IN INDUSTRY:
This research initiative has direct impact on many use cases, including:
Operational and carbon costs of data centers.
Electric Vehicles (EVs), which need high performance at low power consumption to extend vehicle range.
Compute on offshore oil platforms, where connectivity to large data centers is limited. High volume, high speed financial transactions.
Mobile applications that need fast compute on the edge (e.g., autonomous vehicles).
Reducing financial impact of extreme weather events (e.g., by factoring energy costs into operational efficiency).
Lowering energy consumption in telecommunications infrastructure, from data transmission to server operations, to enable the expansion of the digital communications landscape.
Integrating energy-efficient computing in manufacturing processes and robotics to increase efficiency, reduce costs, and minimize environmental impact.
Enhancing energy efficiency in healthcare IT systems and advanced medical imaging techniques, supporting faster, more accurate diagnostics while conserving energy.
Optimizing logistics and supply chain management through energy-efficient computing, reducing the carbon footprint of inventory management, online transactions, and delivery systems.
Reducing the energy demand of content delivery networks and streaming services, addressing the growing carbon footprint of digital media consumption.
TECHNICAL RESEARCH AIMS:
The development of more efficient algorithms: As Moore’s Law comes to an end, designing efficient algorithms that make full use of the available computation becomes an increasingly vibrant area of study. Many of today’s legacy systems depend on algorithms and computer languages that were developed in the days before such limitations and therefore leave potential speed and efficiency on the table. By optimizing computational processes, efficient algorithms reduce processing times, conserve valuable resources, and minimize energy consumption, leading to significant cost savings and a reduced environmental footprint. Moreover, in the context of big data and artificial intelligence, efficient algorithms enable faster data analysis and more accurate predictions, driving innovation and advancements across various domains.
Creating systems and architecture that maximize available computing power: Also relating to the end of end of Moore’s Law, the architecture that current legacy systems depend on is often based on designs that did not prioritize low energy consumption and making the most effective use of resources. By leveraging concepts like parallel processing, load balancing, and distributed computing, developers can fully harness the power of current processors and GPUs.
Rethinking the traditional way cloud computations operate: Cloud computing has been an enormous boon to the economy, but the pressure on companies to have data immediately accessible via cloud is both expensive and currently unsustainable. To support the convenience and innovation of cloud computing while addressing its setbacks, researchers are investigating key topics like applying machine learning to foresee and prevent wasteful cloud outages and more effectively manage limited resources.
Adapting current models for alternative uses: Knowing the monetary cost and energy footprint of programs such as large language models (LLMs), a natural source of inquiry is whether or not previously trained models can be adapted for purposes they were not initially intended or designed for. Early research in this area shows promise in, for example, few- or zero-shot training of LLMs in specialized domains, offering an alternative to companies looking to fill an ML need without the price tag of creating a large model for that exclusive purpose.
Exploring new, compact, and sustainable models: Another option being explored in ML research is the creation of smaller, more targeted, and less expensive models. Rather than training an enormous LLM like ChatGPT, some situations can be better served by compact versions trained on very specific, curated data, making them perfectly targeted for a given use, easier to create, and more sustainable to run than their large counterparts.
CPU efficiency and re-envisioning data centers: Datacenters are the foundation of many areas of computer science, but they are energy-hungry, inefficiently designed, and current operating systems often struggle to keep up with increasing demand. Recent work redesigning datacenters could help with methods such as proactive program scheduling, resource management, and better storage hardware.
LEADERSHIP & GOVERNANCE:
SustainableML@CSAIL will be led by MIT Professors Adam Belay and Charles Leiserson.
Adam’s research focuses on operating systems, runtime systems, and distributed systems. In particular, he builds systems that make datacenters faster, more efficient, and more secure. Charles leads CSAIL’s Supertech Research Group, which investigates the technologies that support scalable high-performance computing, including hardware, software, and theory. The goal is to make scalable computing simpler, faster, and more effective.
Administratively, SustainableML@CSAIL will be run by CSAIL Alliances and will interface with research groups across CSAIL to facilitate research projects poised to generate long-term impact in the world in the space of sustainable computing.
Industry members of SustainableML@CSAIL will each have one seat on the Executive Board along with the faculty director and the Executive Director from CSAIL Alliances. The board will meet at various times throughout the year to discuss research and challenges faced by members. Problem statements will be circulated broadly across the lab to all research disciplines.
There will be an annual meeting on the projects undertaken in this initiative for initiative members and interested community members. SustainableML@CSAIL will also have a session at the CSAIL Alliances Annual Meeting.
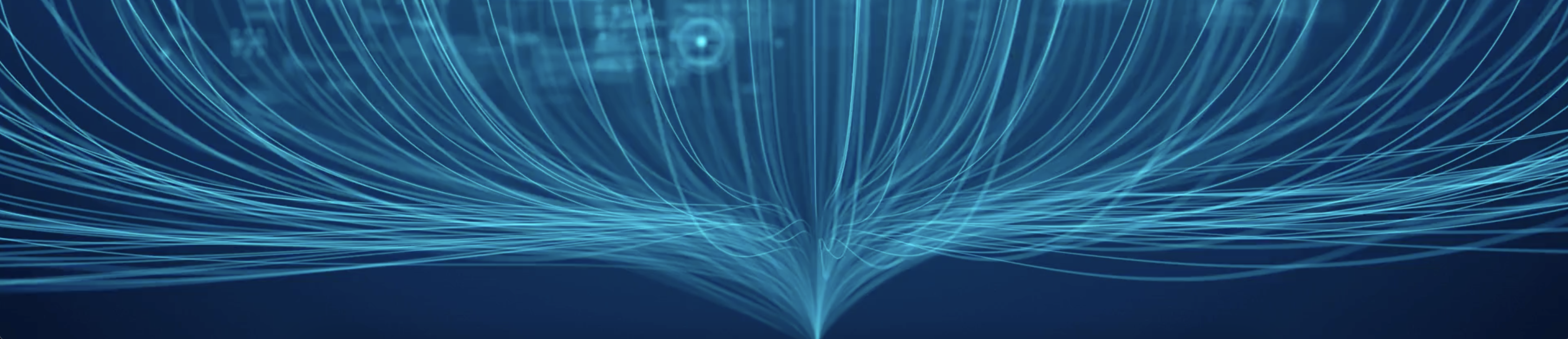